Zachariah Marrero
Ph.D. Student
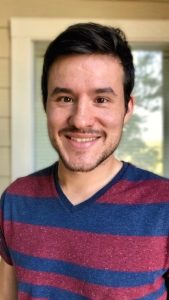
CONTACT INFO
E-mail: zachariah.marrero@gmail.com
RESEARCH INTERESTS:
The goal of Zachariah Marrero’s research is to learn about the nature of everyday human experience by embracing its complexity. His approach to psychological research adopts the breadth and generality of information theory’s view that information is a quantifiable ‘substance’ with a set of inferable properties, to treat thoughts, feelings and behaviors as multiply-determined, informational manifestations of high-dimensional processes. Consequently, his work is interdisciplinary in nature. It seeks to strike a balance between tensions of the explanatory focus traditionally pursued within psychology and providing a full account of a phenomenon. Whereas explanation is generally pursued by leveraging sound theory to reveal simple structure, Zachariah’s work pursues explanation by investing in ecologically sensitive data collection and empirical observations of data models built from flexible machine learning techniques.
For example, he has coordinated and collaborated on large-scale, longitudinal mobile-sensing data collection efforts with the aim of, as directly as possible, confirming inferences from psychological theory on well-being and personality. One project from this effort sought to evaluate the predictive potential of real-world behavior for estimating self-reported depression symptom severity. This project found that the manifestation of depression in real-world behavior is far more heterogeneous than would be expected based on the extant literature where between subjects, fixed-effects models have been the norm. In fact, out-of-sample predictions with fixed-effects models were no better than chance. Consequently, it may be the case that a modeling procedure with greater predictive potential, and therefore greater explanatory power, would condition predictions by first incorporating prominent clusters of symptoms within individuals before inferring how depression is manifested in behavior.
In a separate line of investigation, he is also exploring the application of novel statistical methods to questions that were previously neglected or once presumed to be settled. For example, a common inference about trait scores based on ideas from classical measurement theory is that trait scores should be more predictive than the individual item scores comprising them because the error components of measurements are expected to be random and should therefore cancel out when aggregating items. Zachariah found that while it seems to be the case that traits scores are more efficient predictors, as they are relied upon more frequently than any individual item in a model which incorporates both, machine learning algorithms are able to make better predictions from the set of unaggregated items that comprise the trait scores. This finding suggests that when aggregating, some non-error information is lost. It also raises important further questions about what the boundary conditions are for the theoretical idea that the whole is greater than the sum of the parts.